Picture this: You’ve worked hard to get those leads. You’ve catered marketing campaigns, worked relentlessly on your social media, used every trick your LinkedIn management has to offer, even went for an email outreach – and now the result is there – a significant potential of customers sit in front of you.
But not all leads are created equal, as the proverb says. Some customers are ready to buy your stuff right now, while others might still be in the early stages of curiosity. How do you know who to focus on?
Here’s where lead scoring models become handy. And these are no sales tricks. They represent a data-driven method to understanding your leads and prioritizing those most likely to convert.
The best part? When you use them effectively, lead scoring can revolutionize how you approach sales, transforming chaos into clarity and scattershot efforts into laser-focused precision.
The Basics of Lead Scoring
This is how lead scoring works: it’s a system that assigns a numerical value to each lead, based on various attributes and behaviors. The idea is to quantify the likelihood that a lead will become a paying customer. These attributes could include:
- demographic information (like company size or industry),
- engagement metrics (how often a lead has visited your website).
Therefore, lead scoring is an efficient way to sort your leads out. You probably won’t have the capacity to chase all leads equally. This way you can prioritize your best opportunities by providing an objective framework for your sales and marketing teams to follow.
But here’s where it gets interesting. Sure, a lead who downloads your whitepaper might be interested, but is that enough to assume they’re ready to buy?
The magic happens when you combine data points to tell a fuller story about where the lead is on their journey and what they need to cross the finish line – to buy your product.
Building a Lead Scoring Model
Creating a lead scoring model can seem daunting, but at its heart, it’s about using data to separate wheat from chaff. So how do you build a lead scoring model that works? It all starts with understanding which data points matter most to your business. After all, much like staying on top of the most important tax deadlines ensures your business avoids costly penalties, prioritizing the right data points in your lead scoring model can save your team time and drive better results.
We will highlight three types of data that are critical for any well-crafted lead scoring model:
- Demographic Data,
- Behavioral Data,
- Engagement Data.
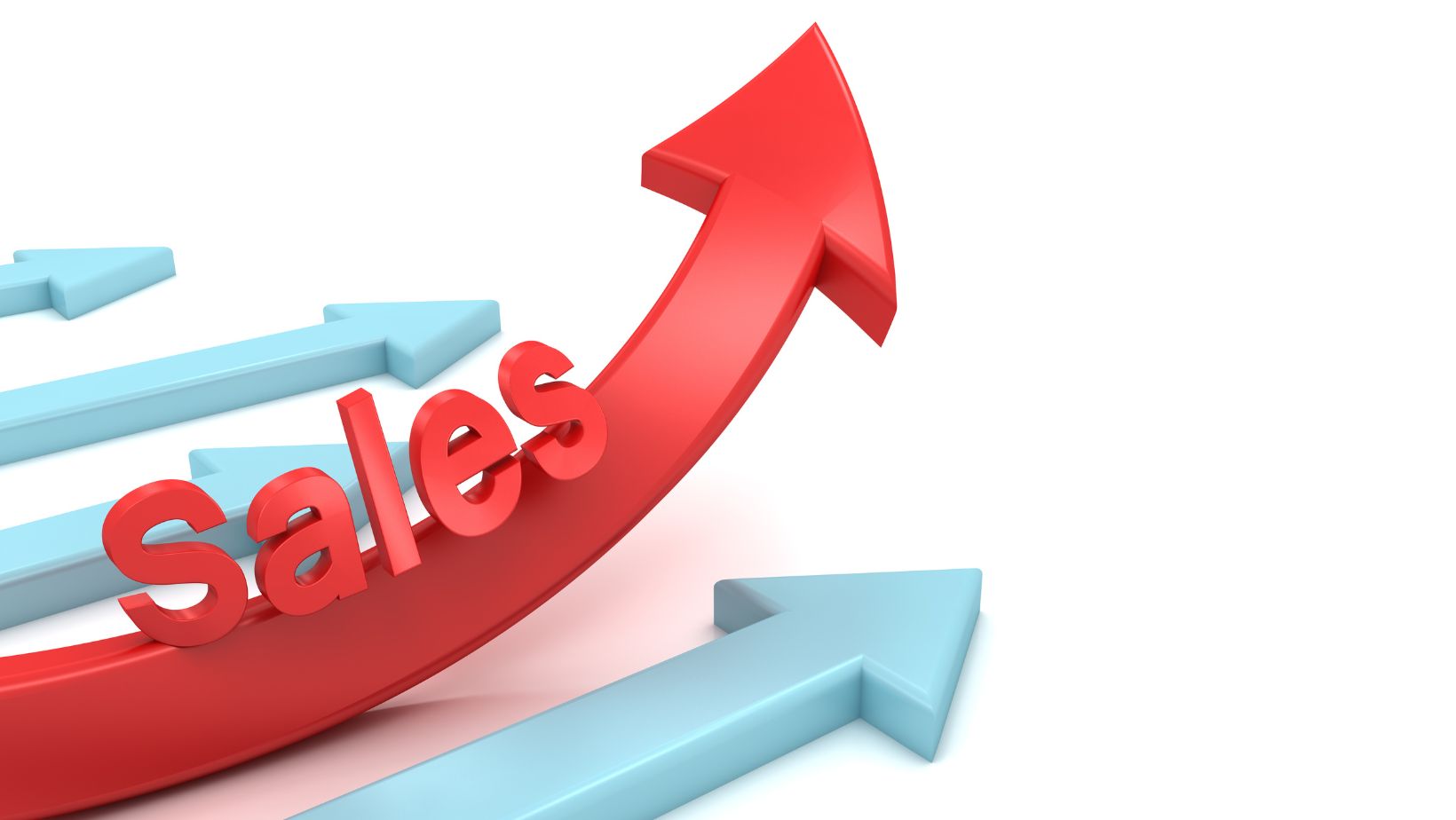
Demographic Data: This is the bread-and-butter of most lead scoring systems. Demographic information includes factors like a lead’s:
- job title, industry,
- company size,
- location.
These details can help you determine whether the lead fits your ideal customer profile (ICP). For example, if you sell enterprise software, you’ll want to prioritize leads that work at companies with many employees, rather than focusing on small startups.
Behavioral Data: This is where things get interesting. Behavioral data tracks the actions a lead takes during their interactions with your brand:
- Did they visit your pricing page?
- Have they clicked on multiple blog posts about a specific topic?
- Did they download a case study?
All of these behaviors are clues about the lead’s interest level. The more engaged a lead proves to be, the higher it should rank on your scoring model.
Engagement Data: This data focuses specifically on how a lead interacts with your marketing campaigns:
- email open rates,
- click-through rates,
- social media engagement.
For instance, a lead who consistently engages with your email campaigns may be further along in the buyer’s journey than someone who has never clicked a link.
Predictive Models
Now let’s kick it up a notch. We have traditional lead scoring models that rely on rules set by humans. However, the real power of lead scoring emerges when you introduce predictive analytics into the equation.
Predictive lead scoring uses machine learning to analyze large pools of historical data and identify patterns that signal a high likelihood of conversion.
In a predictive model, instead of assigning points manually, an algorithm does the heavy lifting. It evaluates every piece of data available, from demographic information to behavioral patterns, and assigns scores based on what has worked in the past.
What’s more, these models adapt over time, improving their accuracy. As more data flows in, the more data you analyze, the greater the likelihood of the model’s predictive value.
These models are more likely to be used by larger businesses with extensive data sets. But even small businesses can work their way around and reach these insights through more accessible tools.
Manual Labor and Lead Scoring Models
So, how do you ensure that your lead scoring model is truly working for your company, giving you useful and valuable data? First, remember that it’s not a set-it-and-forget-it tool.
Regularly review your scoring criteria to ensure they reflect current buyer behaviors and market conditions. Especially if you’re not using the advanced predictive model.
Second, work closely with both marketing and sales teams to refine the model. Marketing teams can feed the system with behavioral data, while sales can provide invaluable feedback on the quality of the leads.
What comes in, must come out. Your job is to try different inputs and control the adequacy of outputs.
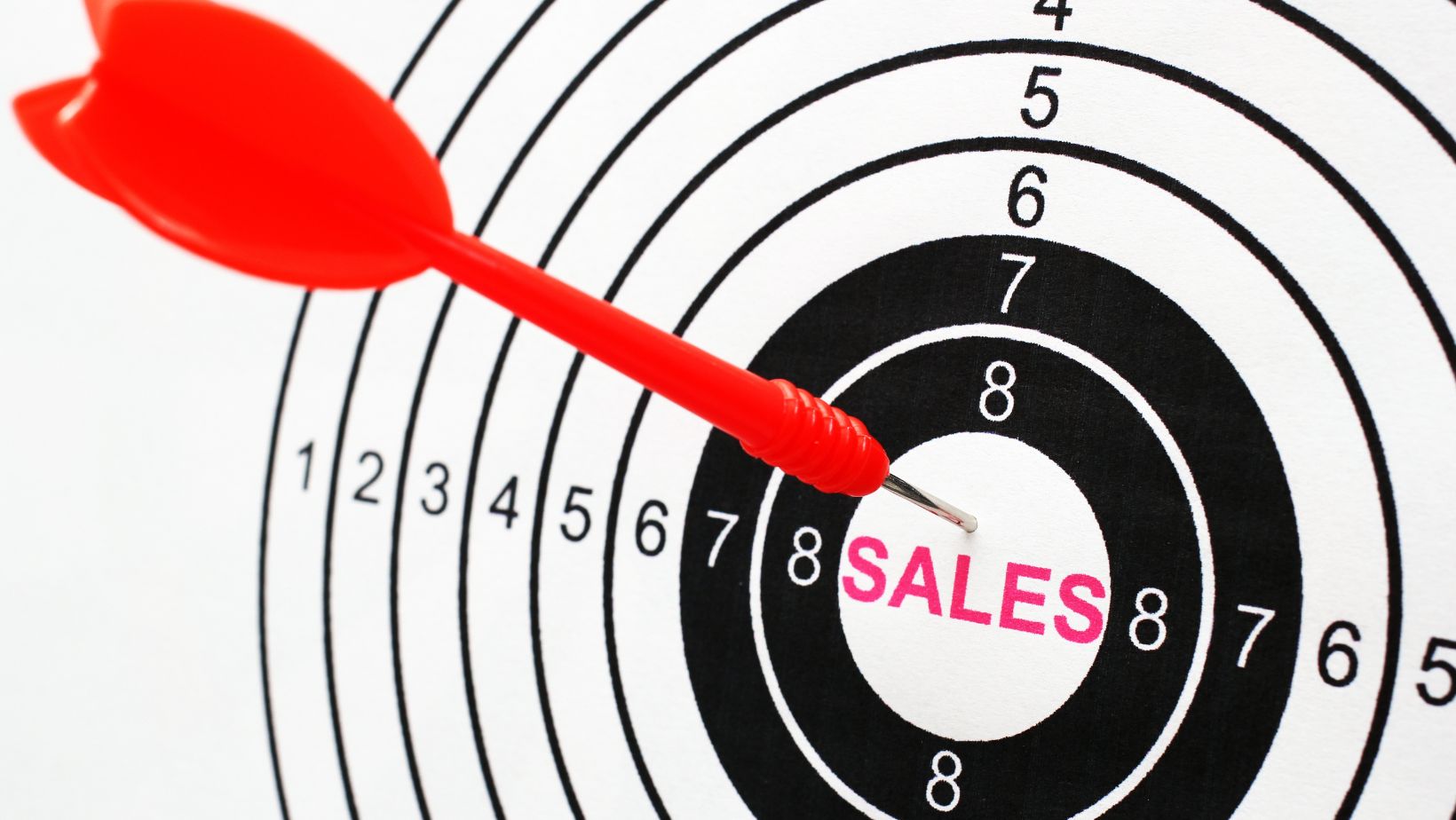
Lead scoring is more than just a tool; it’s a strategy and a prism through which to see the possibilities for your company. It may turn your sales pipeline from a disorganized mess of leads into a well-oiled machine that continuously produces results if carefully constructed and maintained.
By focusing on the leads that matter most, you can unlock sales potential you didn’t even realize was there.